Incorporating Knowledge into your Process Automation
Blog
The World Economic Forum estimated that at the dawn of 2020 there were 44 zettabytes of data in the world. While 44 with 21 zeros is too large to comprehend, you definitely appreciate the velocity of data growth in your organization and the challenge of scaling your processes to produce value for your company.
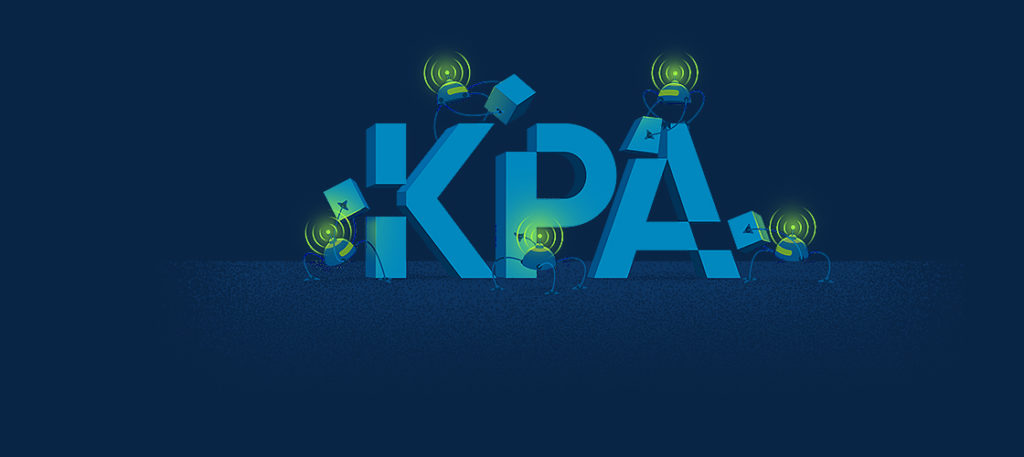
I spent many years in capital markets managing the inherent risk of my data sets that were critical to revenue and risk streams spanning the bank’s global businesses. Through heroic efforts of my team, they found creative ways to apply many different pre-packaged process automations to our ever-complicating data sets. I also saw first-hand how disruptive it could be when an experienced team member leaves, taking with her years of unwritten tribal knowledge. As the team tried to document these nuances of knowledge, their efforts resulted in volumes of edge case scenarios that could not be incorporated into existing automation solutions. To effectively meet all business outcomes, knowledge must be applied and not just reliance on following sets of immutable resolution steps. Each day surfaced slightly different scenarios that had to be reactively addressed, which kept our best minds distracted from creating new valuable knowledge. We needed a smarter solution.
What is Process Automation?
Process automation is packaged as a spectrum of user configured and maintained solutions such as Excel templates, macros, and recently, robotic process automation (RPA). These solutions enable users to chain micro steps together (example below) to accomplish a larger task, but they are inflexible and break when anything changes in the slightest way. If you can even upload your data, most use cases with anything dynamic require constant human intervention. Finally, each automation package has its own bespoke taxonomy and functional model which requires specialized training to shape your problem and its data into the automation solution. As your data and automated processes expand, the risk from controlling these bespoke automated processes can become just as risky and burdensome as doing it manually.

The final straw is accessing and managing unstructured data. Often it cannot be mined by current process automation solutions at scale from your sources. If it can be mined, the slightest change can leave an automation tool dumbfounded.
Knowledge Process Automation?
The DeepSee Knowledge Process Automation (KPA) flows start with desired and scalable business outcomes instead of collections of minute capabilities that the user needs to learn to connect. KPA users achieve these outcomes by loading their unstructured data in its natural format and recording their existing extraction logic with our intuitive UI. Our KPA packages unlock unstructured data that had been completely out of scope for other automation efforts, by incorporating knowledge-based redundancy that humans cannot scale, and that automation bots could not replicate. KPA accomplishes all of this without imposing a strong learning curve that forces you to bend your data and process to “its way of doing things.”
An example solution is extract-to-digitize, which transforms a user’s unstructured data buried deep in your documents into a structured output ready for action. A significant amount of technology powers this solution such as AI classification models, Tesseract, and OCR engines. However, only our applications and the proud engineers who built them need to know that level of detail. Instead, we package a unique experience that feels familiar to the data process expert, and gives them the scalable result they would produce themselves if they conducted the same review manually.
An example screenshot looks like this where we upload one or more docs, highlight what should be extracted on similar docs going forward, and add any required evaluation logic:
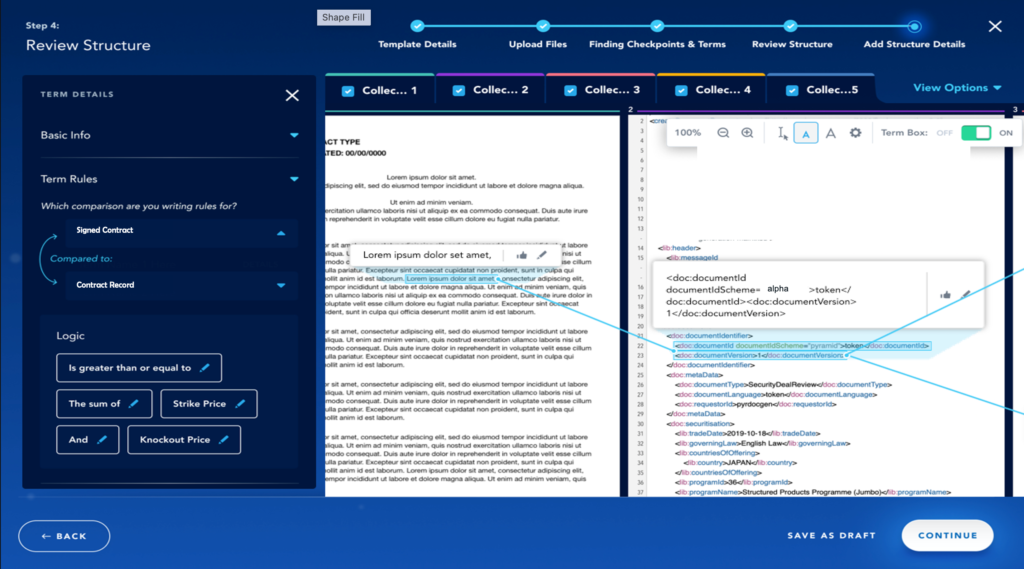
Additional packaged flows can build upon these results seamlessly without asking the user to chain together more micro steps. An example is the term-to- variance solution which calculates the standard wording of a term. Once a standard is identified, the solution calculates variance from that standard in terms of direct match and implied meaning. These calculations make sure that wording changes are intentional and consistent to prevent unintended words or meaning that could translate to business losses or undetected risk exposure.
This example insight shows how KPA extracts from any unstructured data source, and then can uncover deeper insights from both the source itself, but also from any sized group of similar data sources.
Conclusion
As the amount of data in the world continues to increase at an exponential rate, the automation of the processes that ensure accuracy needs to be automated with greater urgency. Legacy automation tools were packaged to maximize the use cases where they could be applied, but this packaging minimized their resiliency and ability to work with data and processes in their native form.
DeepSee’s KPA solutions start with the desired business outcome and how the team achieves those outcomes today. This results in minimal onboarding effort and logic that is executed as knowledge, and not a bespoke set of micro steps that will break the first time something slightly different is encountered.
Do you have an interesting process that runs from unstructured data that needs to be automated or has an existing automation solution that is generating more frustration than it should? If so, let’s talk about a solution that exceeds your expectations.