Generative AI: Act 2 Requires Specialization
Article
By Steve Shillingford and Armen Sargsyan
A friend of mine recently took me for a ride in his new Telsa. It was a wonderful experience. The design is sleek and modern, the ride was quiet and smooth, and the interior really convinced me that, rather than a car with modern features, it’s really a computer on wheels.
We were in a sparsely populated area of the state, so he took the opportunity to show me a few of his favorite features—zero to 60 in under 3 seconds! The other was a demonstration of the “Fully Self-Driving (FSD) computer capability. The name is misleading. I asked, “So you just sit back and nap?” My friend assured me that was not the case, but the act of monotonous driving, especially highway, was “just a joy with FSD enabled.” The feature definitely takes a load off the manual and minute adjustments required to keep the car on the road, between the painted lanes, the right speed (or 9 mph above), and generalized boredom on trips; he was “Tesla Annoying Owner” (TAO) in his praise. But, he reluctantly said, he would never trust it to “take over completely.”
Why is that?
The sentiment makes sense to me. Control is a big deal for humans. So much so, we’ll accept the illusion, even when we know, we really don’t have it. We were all promised flying autonomous cars when we were young, but somehow, the best we could come up with is disposable scooters and a deceiving feature.
This got me thinking about AI generally, and Generative AI specifically. While I have no opinion on when “artificial general intelligence” arrives (AGI), I think GenAI, will make a significantly positive contribution to the way we interact with our environments, our tools, and each other. Unlike many of the incumbents, I don’t see or accept the fear they’re promoting, and wonder a bit about the old “pulling up the ladder” theory. Either way, like cloud computing and decentralized authority, GenAI will soon become “just another feature,” embedded in everything; from our work, to social media, to transportation. It will become a milepost in the long list of computing improvements. Like fire, the wheel, and a hammer, the adoption of GenAI will undoubtedly improve our lives in the aggregate, notwithstanding the edge cases for abuse.
Take search as an example—where an (versus the) answer is available in an instant, and having endless access to information, content, and context does not necessarily mean we’ll see anything other than more noise drowning out the signal. Did it help us navigate “infinity” levels of information? Of course it did. But did it solve the problem all humans crave; “tell me what the answer is.” No, it did not.
Interestingly, there’s a reason why humans—aka carbon-based lifeforms—continue to reign supreme: It’s because of our ability to “reason” through these types of noisy clusters. Why is that? I’m not sure, but I know it has something to do with an adaptive capacity, honed through billions of years of micro-battles for survival, evidenced by the Black Swan outcomes we each represent.
And it is exactly why AI, AGI, and GenAI all represent interesting, but arguably non-threatening, evolutions in a decades-long journey to make machines work “faster, better, cheaper” on our behalf.
From Startups to Fortune 500 companies, businesses are always looking for a wedge to deliver that productivity, increased performance, and reduced risk across their organizations. They all strive to “reason” their way to profits and competitive advantages. GenAI is the latest craze in that journey. Cloud made Amazon a goliath because it brought general purpose, previously very expensive compute to the thousands of companies that couldn’t other afford it. Blockchain put Coinbase on the map by abstracting away the complexity of cryptocurrency. There will be a similar set of winners in the GenAI space. And I would submit it will be the applications that leverage GenAI as a default part of their solution to real business problems.
Generative AI as a catalyst:
With the advent of large language models (LLM) and pervasive adoption of LLM-powered applications in the consumer space, organizations everywhere are trying to make sense of what LLMs can offer while making intelligent decisions about how to use the content these models generate. This field and its associated technology are often termed “generative AI” given the promise of its “net new” content. Most consumers and some forward-leaning enterprises leverage these models in applications powered by “GPTs” (or generative pre-trained transformers, where transformers are the machine learning models powering the LLMs). These apps can be chatbots that focus on specific verticals and offer aggregated results across many content stores or help humans think about the “next question” and pro-actively offer these through the app interface. (E.g., Looking for great Thai food in a city you’re in, a ChatGPT bot could offer to not only give you highly rated results, but offer windows of time where reservations are available, and subsequential automatically book the reservation. All, off the initial ask “What are the top Thai restaurants near me…”)
With GenAI’s ability to generate new content and simplify time-consuming tasks, the technology has broad applicability across multiple industries where content is pervasive, processes are manual, and visibility is low. I remember when touch typing was a critical skillset to learn in the early years of computing… you had to have a minimum of “50 WPM” just to qualify for a job and “typing” as a course was offered in high schools and colleges. Point-and-click followed the invention of hyper text transfer protocol (HTTP), and while typing became less of a concern, search engines became a critical component to any site and any application. While typing was less of an issue, a query format became the difference between highly relevant search returns and a lost afternoon trying to find what you wanted.
So, what does all this mean? And how can we prepare for what comes next… here’s our take.
Prompt engineering is now a must-have skill:
In the world of GenAi, “Prompt Engineering” is going to have a similar effect, as a must-have skillset and driver of productivity in the GPT-driven digital age. However, its potential extends far beyond the realm of consumer-facing applications, supercharged research gathering co-pilots and content production novelty apps. Executives in highly regulated industries are still grappling with how to effectively implement this technology. Due to the cautious and sensitive nature of the work and data managed by Capital Markets firms and other similarly regulated industries, it could take years for them to fully embrace this new wave of innovation, delaying much needed digital transformation.
Specialized applications will drive GenAI adoption in regulated industries:
Today, one of the biggest debates is around how GenAI can be applied to regulated industries. Which industry and process are GenAI models fit for? How do we solve for the problems of GenAI models being confidently wrong on answers it provides (aka hallucinations) in businesses where the answers are deterministic; it’s either a 1 or a 0. These applications must solve end-to-end business problems, while supporting the training and deployment of domain-specific models on top of private data sets to orchestrate and automate complex. Human in the loop feedback is a must-have. In regulated environments, you need input from subject-matter experts, and these applications must deliver a user experience that can support the dynamic nature of complex workstreams.
While Generative AI is still in its early stages, the first wave was not specifically designed for regulated applications in complex environments. Instead, we witnessed rapid advancements in foundation models and infrastructure-level developments, paving the way for a new generation of domain-specific Generative AI. This new breed is now being tailored to specific applications, aimed at solving intricate problem sets. For example, in Capital Markets, everything revolves around onboarding new customers, reconciling trades, and settling confirmations.
Model hallucinations is the biggest barrier to adoption in deterministic businesses:
For context, model hallucination is when a machine learning model creates information that isn’t based on the data it was given. This can happen if the model is too focused on the training data or can’t handle new data well. In highly regulated industries, it’s critical to watch for and fix model hallucinations to make sure the model’s predictions are accurate and reliable – governance, risk, compliance, and AI explainability controls need to be default by design, not a nice to have. The next wave of specialized GenAI applications must deliver hallucination-free results.
In the coming year, we anticipate that the rise of Specialized Generative AI will accelerate the development of enterprise-grade applications to deploy faster, better, and cheaper in the most sensitive and complex environments.
For example, Capital Markets, Insurance, and the Public Sector have a similar set of business and technical challenges:
- The need to mine complex unstructured data across people, processes, and systems (HARD)
- The need to make sense of this data – terms, logic, and undocumented knowledge (HARDER)
- The need to add context, map data relationships, and apply to specific use cases (HARDEST)
Challenges of Deploying Generative AI (1st wave) in Regulated Industries:
- Data privacy and security: Regulated industries often deal with sensitive data, such as personal, confidential, financial information. Ensuring the privacy and security of this data is crucial when implementing generative AI. Businesses must comply with strict regulatory standards to protect their customers’ data.
- Regulatory compliance: Regulated industries are subject to strict regulations and standards. Implementing generative AI in these industries requires compliance with these regulations, which can be complex and time-consuming. Businesses must ensure that their use of generative AI is in line with the relevant regulations and standards.
- Explainability and observability: The use of generative AI in regulated industries must be transparent and explainable. Businesses must be able to explain how their AI systems make decisions and generate outputs. This is important for building trust with customers and regulators, and for ensuring that the use of generative AI is compliant.
- Implementing KPIs and success factors: One of the main challenges many organizations face is getting past the noise and hype surrounding AI, while taking theoretical concepts and applying them to real-world applications.
- Integration with existing systems: Implementing generative AI in regulated industries often involves integrating it with existing systems and processes. This can be challenging, as it requires careful planning and coordination to ensure that the integration is seamless and does not disrupt existing operations with internal systems and other vendors.
This is where specialization is needed… (2nd wave of Generative AI)
DeepSee is purpose-built for highly regulated industries. By leveraging proprietary data sets, applying domain-specific models, and enriching data streams with ontological frameworks, executives can take a measured approach to adopting Generative AI for their specific business needs. Executives can gain visibility across information channels, automate complex work, and even make predictions based on data. Specialized Generative AI is particularly relevant for enterprise customers, who often deal with large amounts of data and complex, disjointed processes.
DeepSee accelerates specialized GenAI adoption in regulated markets.
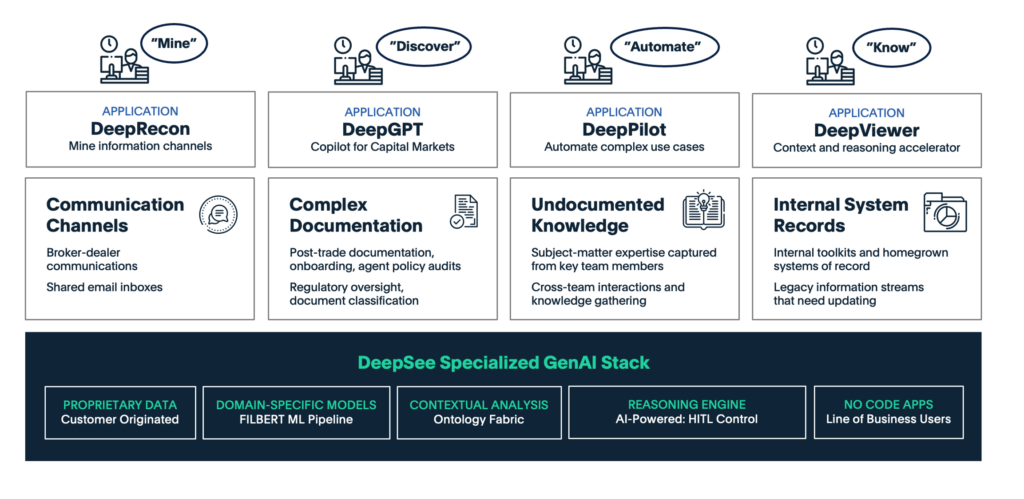
The ability to learn from complex data and improve over time is competitive differentiator. This makes the DeepSee Application Platform an ideal solution for line of business, operations, and technology teams looking for an edge. It’s time to shift our approach from theoretical AI projects to implementing AI for outcomes – optimizing people and work, cutting costs, and gaining operational efficiencies. Schedule a short demo to learn more about how DeepSee is leveraging Specialized GenAI to transform highly regulated markets.