Demystifying the Machine: AI Transparency and Explainability
Blog
By Aubrey Merchant-Dest
Artificial intelligence (AI) is rapidly transforming industries, with finance being at the forefront. From fraud detection to algorithmic trading, AI is making waves. But with great power comes great responsibility, and in the realm of finance, trust and risk management is paramount. This is where AI transparency and explainability (XAI) come into play.
What is AI Transparency and Explainability?
Imagine a black box that takes in financial data and spits out loan decisions. This is essentially how some AI systems operate. Transparency, in this context, is about shedding light on the inner workings of the system. It involves disclosing:
- The type of AI model used: Is it a decision tree, a neural network, or something else?
- The data the model is trained on: Where does the data come from? Is it representative of the real world?
- The model’s decision-making process: How does the model arrive at its conclusions?
Explainability goes a step further. It focuses on making the AI’s rationale understandable to humans, even those without a technical background. This could involve:
- Highlighting the key factors influencing the decision: Was it the applicant’s credit score, income, or something else?
- Providing explanations in plain language: Instead of technical jargon, use clear and concise language.
- Visualizing the model’s reasoning: Use charts or graphs to show how different factors contribute to the final decision.
Why is XAI Especially Important in Regulated Industries?
Financial institutions operate within a strict regulatory framework. They need to be able to justify their decisions, especially when it comes to loan approvals or denials. Here’s how XAI helps:
- Compliance: Regulatory bodies like the Consumer Financial Protection Bureau (CFPB) are increasingly emphasizing the need for fairness and accountability in AI-driven decision-making. XAI helps ensure compliance with these regulations.
- Fairness and Bias Mitigation: AI models can inherit biases present in the data they are trained on. XAI helps identify and mitigate such biases, promoting fair lending practices.
- Risk Management: By understanding how the AI system arrives at its decisions, financial institutions can better assess and manage potential risks associated with the technology.
- Building Trust: Consumers are increasingly concerned about the “black box” nature of AI. XAI fosters trust by offering transparency into the decision-making process.
Here are some real-world examples of XAI in finance:
- Credit Risk Assessment: Some banks use XAI to explain the factors that contribute to a customer’s credit risk score. This helps customers understand why they were approved or denied for a loan, and what they can do to improve their creditworthiness.
- Fraud Detection: Financial institutions use XAI to explain why a transaction was flagged as potentially fraudulent. This helps investigators understand the reasoning behind the AI’s decision and take appropriate action.
- Investment Management: Some investment firms use XAI to explain the rationale behind their AI-driven investment decisions. This helps investors understand the factors that influenced the AI’s decision and make informed investment choices.
Best Practices for XAI in Financial Services:
As XAI continues to evolve, here are some best practices for financial institutions in 2024:
- Focus on Human-in-the-Loop Systems: Don’t let AI make unilateral decisions. Integrate human expertise into the loop to review and potentially override AI recommendations, especially in high-stakes situations.
- Leverage Explainable AI Techniques: Explore techniques like decision trees or rule-based models, which are inherently more interpretable than complex neural networks.
- Invest in Explainable AI Tools: Several vendors offer XAI tools specifically designed to help understand and explain the inner workings of AI models.
- Develop Explainable AI Policies: Establish clear policies around XAI practices within your organization. This includes defining the level of explainability required for different types of AI applications.
The Future: Enter the AI Nutrition Label
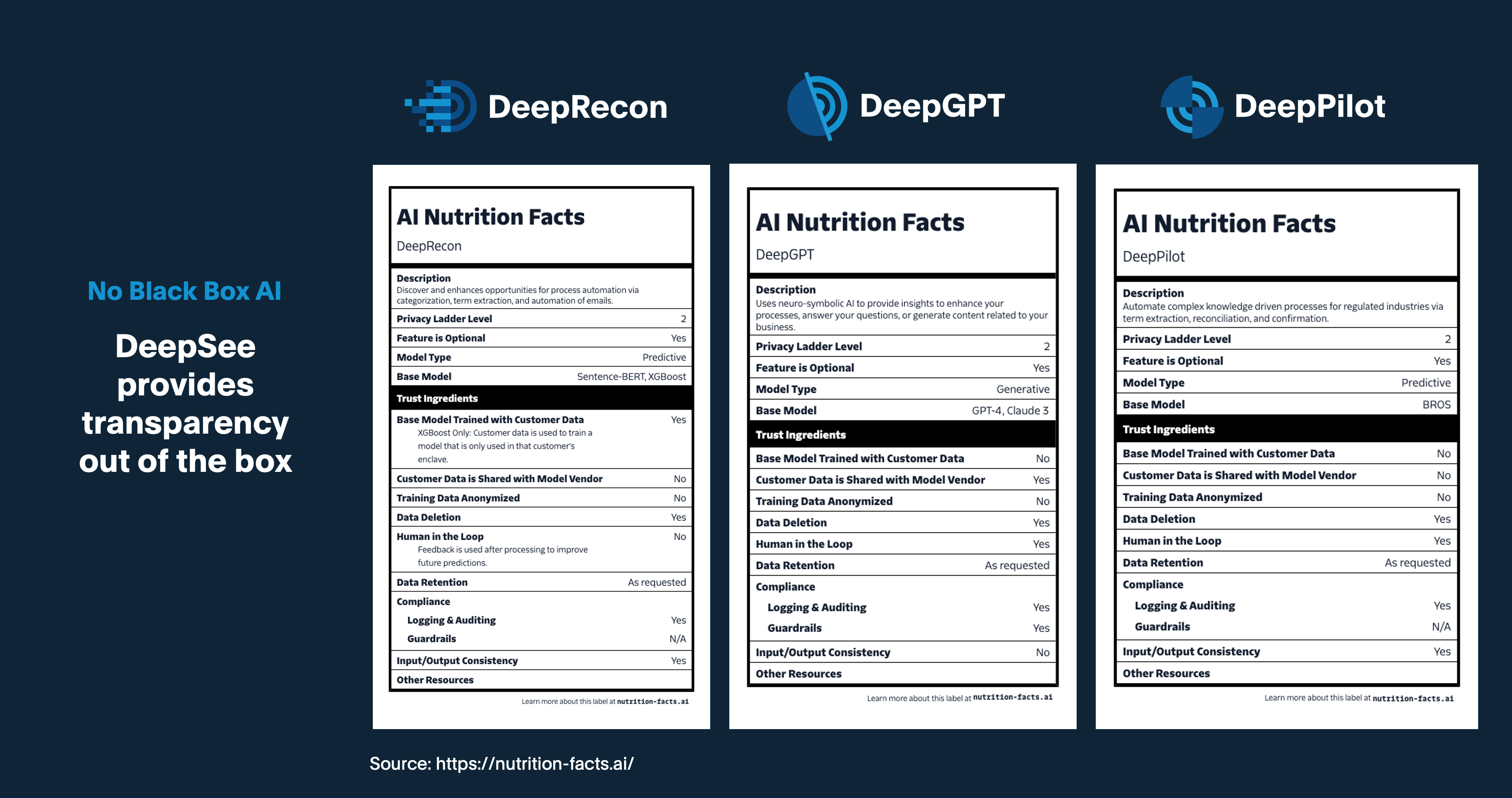
Imagine an “AI nutrition label” for financial products powered by XAI. This label would disclose key information about the AI model used, the data it’s trained on, and how it arrives at its decisions. This would empower both businesses and consumers to understand the rationale behind loan approvals or denials and make informed financial decisions.
While AI nutrition labels are still in their nascent stage, they represent a promising step towards a future where AI operates with greater transparency and accountability. Looking ahead, we can expect further advancements in XAI techniques, making it easier to explain even the most complex AI models. Additionally, regulations around XAI are likely to evolve, mandating a certain level of transparency for AI-powered financial products.
In conclusion, AI transparency and explainability are critical for building trust and ensuring responsible AI development in the financial sector. By embracing XAI best practices and exploring innovative solutions like AI nutrition labels, financial institutions can ensure that AI is a force for good, driving financial inclusion and empowering consumers.