AI Agents Usher in a New SaaS Era: Welcome to Service-as-a-Software
Blog
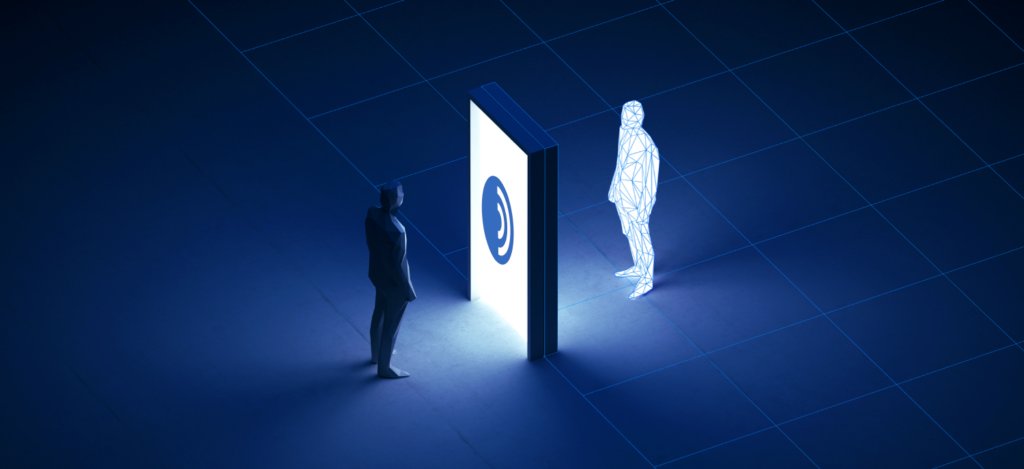
By Armen Sargsyan
I’ve had a front-row seat to the transformative potential of artificial intelligence across various sectors. However, the landscape of AI implementation is far from uniform – especially in the financial services sector where there is no silver bullet approach. It’s a combination:
- In-house DIY toolkits
- Multiple specialized SaaS vendor stacks
- And a good mix of professional services giants in the mix
This combination is highly bespoke. And AI Agents are about to change that.
There’s a significant difference between bespoke AI projects and production AI systems. The world of Software-as-a-Service (SaaS) is undergoing a seismic shift, thanks to the advent of AI agents. This shift is transforming how businesses approach AI implementation, making it more accessible, efficient, and impactful than ever before.
The Limitations of Traditional SaaS
Traditional SaaS models have served us well, but they often fall short in complex, highly regulated environments. These models can struggle to adapt to the intricate needs of industries like finance, insurance, and legal, where compliance and precision go hand in hand. Customizing these SaaS solutions to meet specific customer needs can be both time-consuming, costly, and ripe with technology gaps. Here’s a quick rundown:
Standardization Challenges
Heavily regulated industries require strict regulatory compliance, making it tough to establish a universal approach for defining, scoping, and deploying AI projects. Determining project scope and setting Key Performance Indicators (KPIs) for AI systems are complicated, creating significant barriers to moving AI from the lab to production.
Stakeholder Complexity
AI projects often involve stakeholders from different teams and with differing priorities, making it difficult to define project scope. Achieving consensus on the problem, implementation approach, and scaling initiatives across competing interests is a major hurdle. This lack of alignment can stall projects and hinder the potential benefits of AI.
Quality and Reliability Issues
Bespoke AI systems often suffer from quality gaps, producing inconsistent results and being prone to “hallucinations”—instances where the AI generates information not based on input data. These issues undermine trust and limit the applicability of AI in critical scenarios.
Cost and Resource Intensity
Developing and maintaining bespoke AI systems is extremely costly and resource intensive. The expenses for scoping, implementing, and deploying AI can often outweigh the potential gains, making bespoke AI an impractical choice for many organizations, especially those with tight budgets.
Compliance and Governance Challenges
Ensuring compliance, governance, and AI explainability in bespoke AI systems is particularly challenging. These systems often involve complex, multi-step processes that complicate the establishment of robust governance frameworks. Meeting regulatory requirements and conducting effective audits are significant hurdles in bespoke AI implementations.
So, what is Service-as-a-Software? Why Now?
Service-as-a-Software flips the traditional SaaS model. Instead of providing a one-size-fits-all software solution, S-a-a-S offers a more tailored approach by integrating AI agents into the core of the service. These AI agents are designed to handle multi-step processes, adapt to specific business needs, and seamlessly integrate with existing systems – like workflows. They act as intelligent intermediaries, refining data, automating tasks, and delivering actionable intelligence.
Real-World Applications + Agents = AI That Mirrors Humans
For instance, regulated enterprises face significant hurdles when adopting AI-powered solutions due to their historical reliance on large teams for complex, manual processes, multi-geo compliance requirements, and deterministic nature of their businesses. Before LLMs and Agentic AI, automating intricate regulatory processes end-to-end was done with brittle and bespoke workflow solutions.
AI-powered agents have the potential to automate deterministic, nuanced, and complex work items, enabling regulated enterprises to overhaul legacy systems and gain a significant competitive advantage over their peers. At DeepSee, our view of an AI Agent = a financial use case / process that requires extensive, multi-step functions to orchestrate complex work. Examples: Data Quality, Perpetual KYC, Trade Affirmations, etc.
As we embrace this new SaaS era, businesses can look forward to more intelligent, adaptable, and efficient solutions. AI agents are not just enhancing traditional SaaS models; they are redefining them. Welcome to the future of Service-as-a-Software.